AI-Generated Diet for Pregnant Women
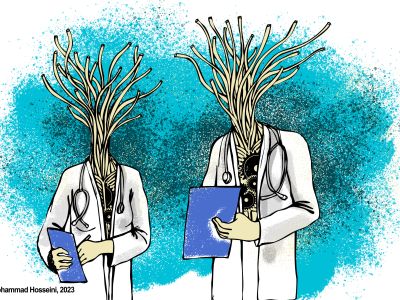
This hypothetical scenario is about a dietician researcher who used a free application based on Large Language Models (DietGPT) to offer a daily diet to pregnant women. Once data collection is completed, the researcher discovers a tangential finding but decides against publication. Two years later, she receives an email from an advocacy group about DietGPT, noting that circumstances of some mothers were not considered in research.
Mandy is a dietician interested in the relationship between sugar intake during pregnancy and preeclampsia (MedlinePlus definition of preeclampsia: “high blood pressure and signs of liver or kidney damage that occur in women after the 20th week of pregnancy”). As part of her new IRB-approved project to study preeclampsia, she uses a free mobile application called DietGPT to engage with research subjects.
Upon consenting to participate in Mandy's study, pregnant women interact with DietGPT to report their daily diet and food preferences. DietGPT aggregates this information with anonymized medical history provided by Mandy, and then uses the existing literature on pregnancy diet to craft a personalized low-sugar meal plan for each participant. Additionally, DietGPT provides weekly reports on patients’ progress to Mandy (including reports of their daily food intake logs), with specific recommendations per patient on how to improve their eating habits to lower blood pressure and decrease the likelihood of preeclampsia. Mandy reviews (and if necessary, edits) these reports before sending them to patients.
After providing suggestions to one cohort (n=100) and following up on their end of pregnancy report, Mandy concludes that the provided suggestions by DietGPT did not decrease the average likelihood of preeclampsia. That said, she noticed a tangential finding: while all the White American mothers (n=78) had a successful at-term birth, some African American (n=4) and Hispanic (n=3) mothers had a preterm birth (high sugar intake is believed to contribute to preterm birth, see here). Mandy decides against publishing a manuscript about this trend based on three reservations:
- Participants consented to join her study on preeclampsia. Given the narrow scope of consent, a formal investigation of this new trend requires modifying the IRB application with new research questions and the need for reconsent, leading to a lengthy process.
- She does not feel confident to write a paper about preterm birth and would have to invite new collaborators. However, since the sample size is small, her results are unlikely to enthuse other researchers to collaborate.
- Since preterm birth was not among her initial hypotheses, she fears about potentially being accused of HARKing (Hypothesizing After the Results Are Known).
Nevertheless, participants did not know about this trend, and those who asked about Mandy’s results (regarding preeclampsia), were told that the suggested diet did not decrease the likelihood of preeclampsia. Soon after, Mandy moved to a different institution and changed her research focus and never published the results.
Two years later, an advocacy group contacts Mandy and other researchers who were among early users of DietGPT but did not publish their results. The advocacy group asked: “Our research shows that DietGPT’s suggestions were harmful to mothers from specific racial groups. Did you notice any relationship between the offered diet and preterm birth in specific racial groups within your sample?” Mandy responds “Exploring preterm birth was not among my research goals. Furthermore, according to my IRB and data management protocol, I had to delete the data one year after collection, and so I cannot respond to queries about that project with certainty because the data is deleted.”
Discussion Questions
- Are there any requirements for using mobile applications in research?
- After being contacted by the advocacy group, Mandy thinks to herself: “It is not my fault that DietGPT suggestions to some participants did not work. Calculating sugar intake and generating daily meal suggestions for all participants were so complicated and time consuming that I could have not replicated them, so there was no way I could have prevented this.” Is she right to think so?
- When researchers use mobile applications, who is responsible for harmful suggestions and conclusions and why? What could Mandy have done to mitigate risks?
- Suppose the advocacy group determines that DietGPT has provided culturally insensitive suggestions, leading to their dismissal by non-White American mothers. How might this conclusion influence our perspective on the adequacy of Mandy's research design in ensuring participants’ safety?
- Non-publication of results (so-called file drawer problem) is unethical and considered wasteful (see here). However, Mandy’s findings were tangential to her main research question and so, this is not a clearcut case. What would you do if you were in Mandy’s shoes?
- Mandy has three reasons for not wanting to publish her results. Are her concerns valid?
- The case indicates that Mandy has not lied. Even in her response to the advocacy group, she dodged the question without lying per se. Can you name any flouted moral principles other than honesty and highlight their significance for research?
- If DietGPT’s suggestions had positively decreased the likelihood of preeclampsia, Mandy would publish results. What would be the ethical way to acknowledge DietGPT’s contribution?
- Can you think of any ethical norms that mandate Mandy to publish her tangential results about preterm birth?
- Can you think of any ethical norms that mandate Mandy to communicate her tangential results about preterm birth to participants? White American mothers were not negatively affected. Should they also be informed?
This work was supported by the National Center for Advancing Translational Sciences (NCATS, UL1TR001422), National Institutes of Health (NIH). The funders have not played a role in the design, analysis, decision to publish, or preparation of the manuscript. This work does not represent the views of the NCATS, NIH, or U.S. government.
As the use of artificial intelligence applications based on Large Language Models (e.g., ChatGPT and Elicit) becomes prevalent in research, discussing possible benefits and shortcomings is crucial. In this scenario, DietGPT combines subjects’ anonymized medical information and food intake with insights from existing literature to offer tailored dietary recommendations. While this is a fictitious scenario, chatbots have been used to offer dietary advice. For example, in February 2022, The National Eating Disorders Association (NEDA) launched its chatbot, called “Tessa,” to offer advice to people seeking help for eating disorders. NEDA disabled Tessa in May 2023 after some complained about receiving harmful advice (more here).
The case prompts researchers to reflect on using software in research, modifying IRB applications, and dealing with accidental findings. By wrestling with these issues, researchers will be better prepared to navigate similar situations in their engagement with software applications and will develop a deeper understanding of involved challenges.
Below are discussion tips for the discussion questions.
Researchers might consider referring to relevant directives provided by their IRB, highlight Terms of Service, User Agreements, and liability limitations of mobile applications, and consider FDA suggestions on using mobile medical applications.
Researchers could discuss alternatives to using DietGPT and emphasize Mandy's due diligence duty (e.g., read reports and reviews about DietGPT, enquire colleague’s views, test DietGPT and compare its conclusions with similar applications).
Researchers could refer to the lack of agency and consciousness of tools to stress that when employing any software or mobile application, users are ultimately responsible. Furthermore, researchers could emphasize Mandy's due diligence duty to mitigate risks.
Researchers could discuss Mandy’s responsibility to recognize and anticipate DietGPT's limitations and their timely communication to participants. For example, Mandy could have incorporated various strategies to her research protocol to evaluate how closely subjects followed DietGPT's suggested meal plans (e.g., using regular check-ins and surveys).
Researchers could highlight the possibility for alternative publication outlets that encourage publishing unexpected results with the aim to create awareness about DietGPT’s limitations. Additionally, reaching out to colleagues who work on preterm birth to seek advice and explore the possibility of larger follow-up studies would be helpful.
Researchers can refer to IRB modification process (also called IRB amendment) and relevant institutional regulations (see e.g., NIH Policy 3014-204). Researchers can share experiences about collaboration with colleagues who have not been involved in a study from the start and challenges of dealing with accidental findings or results that were not based on a hypothesis that was being tested.
Researchers can use various frameworks suggested for ethical conduct of research to identify relevant principles. Examples include the Office of Research Integrity shared values and ethical principles of research offered by David Resnik or those offered by Beauchamp & Childress.
Software applications (including chatbots) should be cited in-text and mentioned among references, but they cannot be authors or be mentioned in the acknowledgements (see here).
Researchers can use various frameworks suggested for ethical conduct of research to identify relevant ethical values and principles. Examples include the Office of Research Integrity shared values and ethical principles of research offered by David Resnik.
Researchers can discuss erosion of society’s trust in science and use various frameworks suggested for ethical conduct of research to identify relevant principles. Examples include the Office of Research Integrity shared values and ethical principles of research offered by David Resnik.