Case: Big Data & Public Health
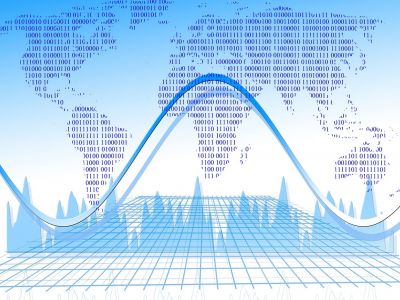
A computer science graduate has an opportunity to work managing large data sets from smartphone applications designed to track individuals' personal health information. His father, an epidemiologist, encourages him to consider the project's use of personal data, as well as the ability to design algorithms that could accurately predict and track outbreaks based on this kind of data.
Zhang Kar-wai, a graduate from Stanford University’s department of computer science, has recently accepted his first paid position at a start-up company in San Francisco. The company’s main products consist of a line of smartphone applications designed to track individuals’ personal health information, including their medical records, their seasonal illnesses, their blood pressure and blood glucose levels, their eating habits, their sleep cycles, and even their weight and reproductive health. The products are designed primarily to help individuals reach health-related goals, and enable users to manage their overall health and wellbeing.
Zhang was hired to join the team responsible for managing the large data sets generated by the users of these apps. One of his responsibilities will be to develop algorithms and analytic tools that can track the outbreak and spread of infectious diseases in real-time using data gathered from individuals using their applications. Their goal is to improve on the traditional methods used by the US’s Center for Disease Control and Prevention (CDC) and the UN’s World Health Organization (WHO).
Zhang loved developing algorithms as a student and he is looking forward to participating in the team’s project. He was excited to tell his father, a professor of epidemiology at Stanford’s School of Medicine, about his assignment. However, when Zhang told his father about his responsibilities at his new job, his father’s reaction was not what he expected. Zhang’s father expressed concern about the project’s use of personal data, as well as their ability to design algorithms that could accurately predict and track outbreaks based on their data.
Zhang explained to his father that the data will be aggregated and the algorithms will undoubtedly sometimes fail, as all models do, but they will be continuously tested and upgraded. So, he assured his father that he was up to the task. His father remained hesitant to share in Zhang’s enthusiasm and warned him to think more about the ethical implications of his project, and not just about whether his algorithms will succeed or not. Should Zhang take his father’s concerns more seriously?
Discussion Questions
- Is it ethically permissible to use data from Internet search engines or applications for national public health purposes? If so, does this mean it is also permissible to use privately collected data in global public health contexts?
- Should users of online applications or search engines be notified about the potential use of their personal data (even in aggregated form) for public health measures? Why/why not?
- What are some of the ethical risks of the proposed big data analytics or algorithms, which can sometimes lead to false positives in their efforts to identify outbreaks and/or predict outbreak trajectories? What might be done to mitigate such risks?
Bibliography
Butler, Declan. “When Google got flu wrong.” Nature 494, no. 7436 (2013): 155.
Brownstein, John S., Clark C. Freifeld, and Lawrence C. Madoff. “Digital disease detection—harnessing the Web for public health surveillance.” New England Journal of Medicine 360, no. 21 (2009): 2153-2157.
Cook, Samantha, Corrie Conrad, Ashley L. Fowlkes, and Matthew H. Mohebbi. “Assessing Google flu trends performance in the United States during the 2009 influenza virus A (H1N1) pandemic.” PloS one 6, no. 8 (2011): e23610.
Ginsberg, Jeremy, Matthew H. Mohebbi, Rajan S. Patel, Lynnette Brammer, Mark S. Smolinski, and Larry Brilliant. “Detecting influenza epidemics using search engine query data.” Nature 457, no. 7232 (2009): 1012-1014.
Ives, Mike. “When Epidemics go Viral.” The Atlantic. October 18, 2016. https://www.theatlantic.com/health/archive/2016/10/when-epidemics-go-viral/504503/. Accessed November 2, 2016.
Lazar, David, and Ryan Kennedy. “What can we learn from the epic failure of Google Flu Trends.” Wired. October 1, 2015. https://www.wired.com/2015/10/can-learn-epic-failure-google-flu-trends/ Accessed November 2, 2016.
Lazer, David, Ryan Kennedy, Gary King, and Alessandro Vespignani. “The parable of Google flu: traps in big data analysis.” Science 343, no. 6176 (2014): 1203-1205.
Mikal, Jude, Samantha Hurst, and Mike Conway. “Ethical issues in using Twitter for population-level depression monitoring: a qualitative study.” BMC medical ethics 17, no. 1 (2016): 1.
Salathe, Marcel, Linus Bengtsson, Todd J. Bodnar, Devon D. Brewer, John S. Brownstein, Caroline Buckee, Ellsworth M. Campbell et al. “Digital epidemiology.” PLoS Comput Biol 8, no. 7 (2012): e1002616.
Vayena, Effy, Marcel Salathé, Lawrence C. Madoff, and John S. Brownstein. “Ethical challenges of big data in public health.” PLoS Comput Biol 11, no. 2 (2015): e1003904.
Vayena, Effy, Anna Mastroianni, and Jeffrey Kahn. “Ethical issues in health research with novel online sources.” American journal of public health 102, no. 12 (2012): 2225-2230.
White, Michael. “The Ethical Risks of Detecting Disease Outbreaks with Big Data.” Pacific Standard. February 24, 2015. https://psmag.com/the-ethical-risks-of-detecting-disease-outbreaks-with-big-data-37ba15345aa7#.5cwzsw7b8. Accessed November 2, 2016.
The author wishes to acknowledge the contributions of Karin Ellison, OEC - Life and Environmental Sciences Editor, and Joseph Herkert, OEC Engineering co-Editor. They provided valuable input in selecting topics and crafting the resources.
The fictional scenario described above is loosely based on a recent initiative by Google. In 2009, research scientists at Google published a study in Nature, describing their methods for tracking seasonal and pandemic influenza outbreaks using data generated from monitoring health-seeking behaviour on Internet search engines (Ginsberg et al. 2009). They had developed tools to track outbreaks in real-time in order to improve upon the traditional methods used by the Center for Disease Control and Prevention (CDC), which take approximately two weeks to gather and analyze data. The algorithms developed by the scientists at Google led to the creation of Google Flu Trends (GFT), a web service launched in 2008 to track flu outbreaks. The service is no longer publishing its results, but its data are made available to other researchers.
The 2009 Nature paper is often used as a paradigm example to illustrate the emergence of a new field referred to as digital epidemiology, or digital disease detection (DDD) (Brownstein et al. 2009; Salathe et al. 2012; Vayena et al. 2015). This field shares the goals and objectives of traditional epidemiology (e.g. public health surveillance, disease outbreak detection, etc.), but makes use of electronic information sources, such as internet search engines, mobile devices, and other social media platforms, which can generate data related to public health but that are not explicitly designed for collecting public health-related data. The motivation behind DDD initiatives, like Global Flu Trends, is to mine large datasets in order to accelerate the process of tracking and responding to outbreaks of infectious diseases.
In 2013, Google’s program to track influenza outbreaks was heavily criticized for mis-estimating the prevalence of influenza outbreaks (Butler 2013, Lazer et al. 2014, Lazer & Kennedy 2015). Its first big mistake occurred in 2009, when it underestimated the Swine Flu (H1N1) pandemic (Butler 2013; White 2015), due to changes in people’s search behaviour with respect to the categories of “influenza complications” and “term for influenza” given the non-typical seasonal outbreak of H1N1 during the summer months (Cook et al. 2011). Then, in 2013, Nature reported that GFT significantly over-estimated outbreaks of influenza (Butler 2013; Lazer et al. 2014). In a comment published in Science in 2014, Lazer et al. reported that GFT had been consistently over-estimating the prevalence of flu outbreaks before then, inaccurately predicting the prevalence of flu cases in 100 of 108 weeks during the 2011-2012 flu seasons (Lazer et al. 2014).
GFT’s track record of mis-estimations has been described as “big data hubris” – “the often implicit assumption that big data are a substitute for, rather than a supplement to, traditional data collection and analysis” (Lazer et al. 2014, 1203). In epidemiology, traditional data collection and analysis involves gathering data from structured interviews, archives, censuses, and surveys, and then to look for patterns and trends in the data. However, most scientists commenting on the case of GFT have insisted that, despite its failures, the use of big data in epidemiology can be extremely valuable for public health surveillance (Lazer et al. 2014, Lazer & Kennedy 2015, White 2015).
The GFT case has invoked many epistemological questions about how to improve Google’s flu algorithms, and big data analytics more generally, and how public health policy and decision-makers ought to use these tools. But, it has also engendered ethical concerns at “the nexus of ethics and methodology” (Vayena et al. 2015).
For example, there can be harmful consequences when such models are woefully inaccurate or imprecise. False identification of outbreaks or inaccurate and imprecise predictions of outbreak trajectories could place undue stress on limited health resources (Vayena et al. 2015). Wrong results or predictions might also undermine the public’s trust in scientific findings, and worse, might lead to the public’s dismissal of public health warnings.
In addition to worries about maintaining the public’s trust on issues of public health, researchers developing models aimed at detecting outbreaks must consider that their results risk harming individuals, businesses, communities, and even entire regions or countries (Vayena et al. 2015). This harm may take the form of stigmatization of groups, and financial loss due to prejudice or restrictions on travel to tourist destinations. It can also restrict the freedom of individuals in the form of imposed travel restrictions or quarantines. Consequently, ethicists have stressed that “methodological robustness” with respect to digital epidemiology is “an ethical, not just a scientific, requirement” (Vayena et al. 2015, 4).
As with other instances of big data collection and use in the life sciences, the use of big data gathered online in social or commercial contexts for public health purposes raises ethical issues about an individual’s right to privacy and notions of informed consent when that data is used for research purposes. However, in this context, it has been suggested that private corporations that have access to relevant data might have a moral obligation to share that data for matters related to public health and public health research. This consideration raises questions about how to regulate private-public partnerships with regards to data ownership within a global context in order to uphold the values of transparency, global justice, and the common good in public health research (Vayena et al. 2015).